This is also the reason why 2025 is being called the "Year of AI Agents." Some models Like large language models, transition from generative models with simple input-to-output structures, the AI agents capable of thinking and interacting with the world.These agents can plan -> attempt -> observe real-world feedback ->evaluate with a reward model -> believe further, and possibly revert to redoor continue. They are more adaptable, continuously thinking, and interacting with the real world in a nonlinear, human-like way. These agents have first emerged in interaction with the virtual world (GUI Agents).

However, as a long-time player in this field, the iMean AI team has continuously invested in this area for three years. Unlike the tech giants that focus on developing pre-trained models, iMean AI provides infrastructure centered around post-training. This is the most unique toolchain and infrastructure in the industry that has reached the level of productization. It has been adopted by mainstream enterprises and academic institutions, such as ServiceNow, and Carnegie Mellon University.

With three years of research in infrastructure and fast and pragmatic engineering iterations, iMean AI’s infrastructure products have accumulated significant strength to quickly meet the industry's most urgent needs amidst the wave of GUI Agent transformation. This has helped iMean AI secure a rare position at the center of the GUI Agent wave.
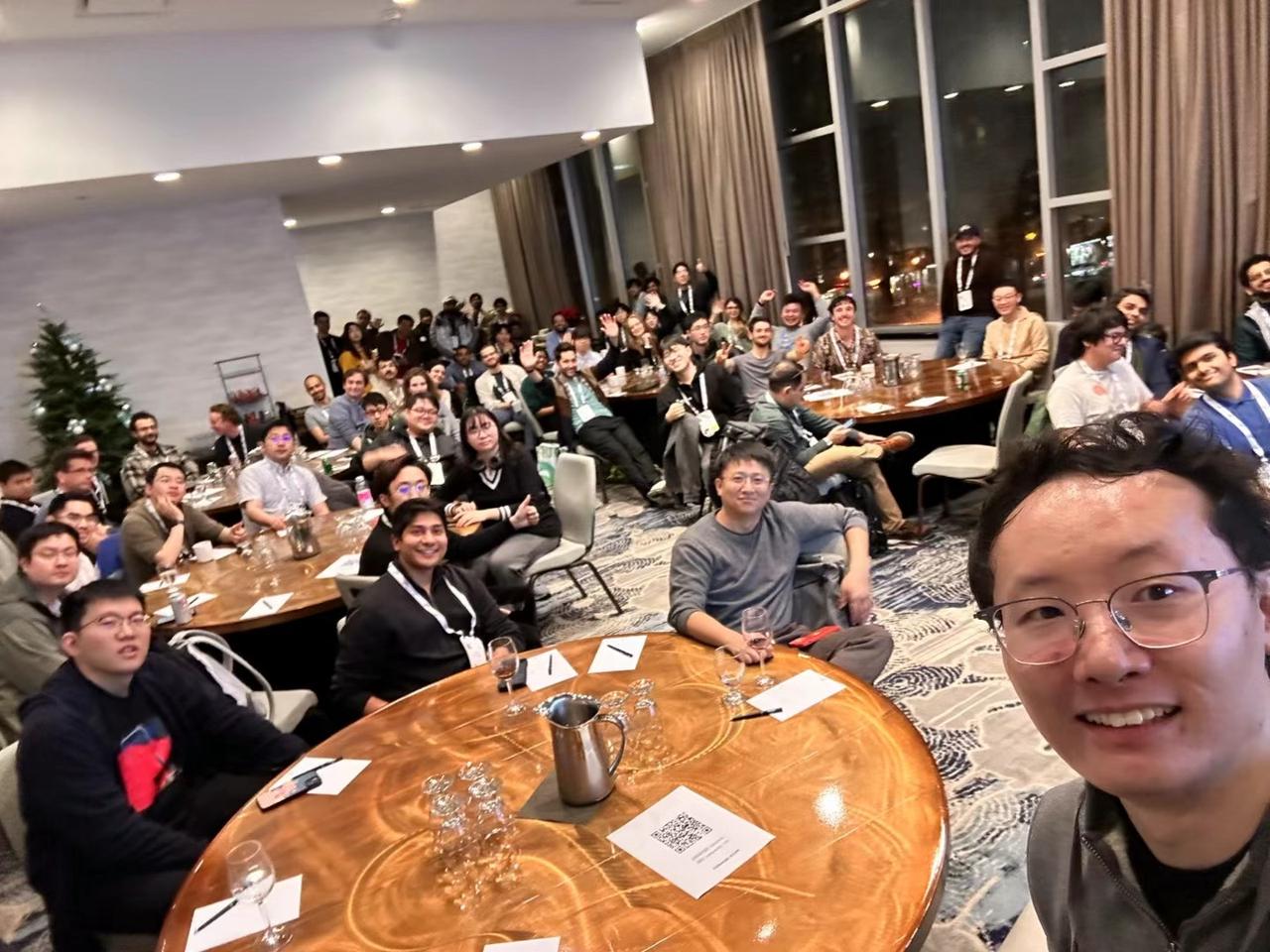
In the technical engineering practice of achieving AGI, post-training is increasingly occupying a more important position, compared to pre-training. At NeurIPS 2024, llya, the former Chief Scientist of OpenAI announced the end of pre-training. The main reason is that computational resources can continue to improve with further optimization of hardware and algorithms. However, the corpus of data used for pre-training is entirely dependent on the accumulation of the past 20 years of internet data, which cannot be generated in large quantities in the short term.

For the GUI Agent, like WebAgent, the scarcity of data itself is a decisive factor in determining its quality. Corpus data has been accumulated over the past two decades through digital traces left by people on the Internet. The training datasets required for WebAgent need to provide a complete, structured description of the world model, action space, reward signals, intentions, and planning, which can almost only be generated by annotation.
Therefore, data is the first key challenge for the post-training of WebAgent that needs to be addressed. The other two core challenges are the WebAgent framework and the benchmarking and reward mechanisms for evaluating WebAgent. Together, these three factors determine WebAgent's scaling law. iMean AI's post-training infrastructure is at the forefront of the industry in these three aspects.
In terms of data, iMean AI Builder is currently the most comprehensive tool for data annotation. It supports the widest action space coverage, the deepest observation space exploration, multi-level process intention support, and the visualization of reward signals for annotation. Additionally, it has solved many long-tail problems in continuous engineering practice. For example, the largest open-source WebAgent dataset, Mind2Web Live, was annotated with the support of iMean AI Builder. Moreover, the company has accumulated a vast amount of closed-source data internally.

In terms of the WebAgent framework, iMeanAI's framework provides key tools related to reinforcement learning, fine-tuning, in-context learning, and other capabilities needed for post-training. This effectively reduces the cost of post-training and enhances the stability of WebAgent outputs. Compared to pre-trained models, which typically can only perform basic capabilities for 5-10 steps, this framework extends the stable output length of action sequences for target tasks to over 500 steps. It enables iMean AI to meet the ability to be the first to land in scenarios with commercial value and has been commercially verified by many Fortunes 500 customers.

As Chief Product Officers at OpenAI and Anthropic, Kevin Weil and Mike Krieger, respectively mentioned, "Current models are not limited by intelligence level but by the evaluation methods." So, evaluation methods are crucial for WebAgent training. Model training is based on correcting deviations between output results and a benchmark. Additionally, the training of reward models also depends on many reward signal annotations relative to the benchmark. A series of good evaluation methods is the only way to scale WebAgent.
In terms of evaluation methods, iMean AI's WebCanvas is the first benchmark that can systematically evaluate WebAgent in real-world environments. Users can host their WebAgent in the WebCanvas, and through evaluation further train and optimize the results of the WebAgent.
